The neuroscience community has been trying to build a brain-to-computer translator, that is, a system that records the electrical chatter of neurons and deciphers the thoughts of the person to whom those neurons belong. With such a BCI system, a paralyzed user could control their computer with thought alone. Its a tough task for many reasons, one of them being not many people are willing have electrodes implanted in their brain to test experimental algorithms, but without willing subjects algorithm design is very challenging for neural engineers.
As a solution, we created a model of invasive BCIs, the joint angle BCI, or jaBCI. The model lets able-bodied subjects generate synthetic neural firing by interacting with an artificial neural network (ANN), which in turn, lets us test and prototype decoders.
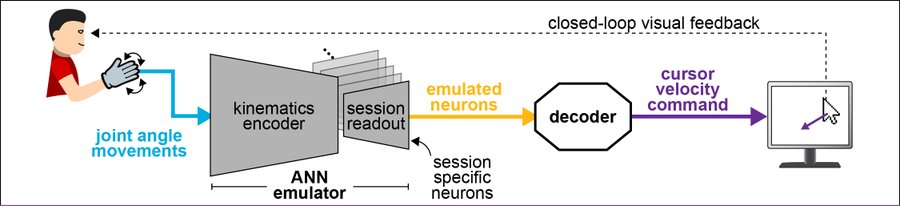
The goal is to overcome sample size limitations inherent to invasive BCI studies in humans and monkeys when assessing neural decoders. With jaBCI, able-bodied subjects can use any decoder in closed-loop, giving us statistically reliable assessments and rapid prototyping.
How does it work? Subjects’ finger joint angles (“ja”BCI) are input to an ANN trained to produce monkey M1 firing. The synthetic firing is decoded in real-time into cursor velocity commands to hit targets. The ANN even simulates different neurons across visits (just like the changing neurons that an BCI has access to each day of use).
Does it work? Yes! To validate, we had subjects (n=25) do the same 4-target center-out task with the same velocity KF decoder as 3 invasive BCI studies did in ~2010. jaBCI users had the same accuracy (% targets missed) and path straightness (ME) across 4 visits as BCI users.
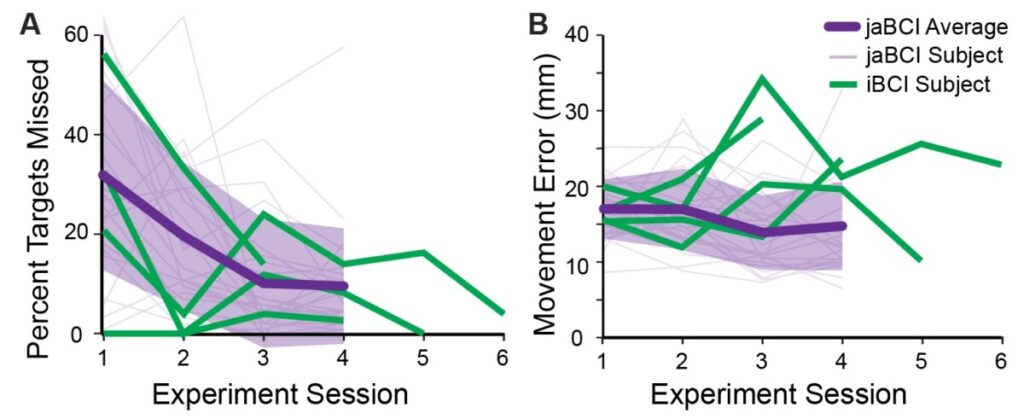
Note the large performance variability of both the jaBCI users (purple) and the invasive BCI users! It may be dubious to draw conclusions about how good a decoder is for the general population from one or two BCI users. Our model can help assess this.
To validate the ANN (that generates the synthetic neural firing), we PCA-projected all neurons through time down to one point per trial. The synthetic data have a low-dim geometry similar to that of the monkey data we trained the ANN with, giving us confidence that the emulated neurons track the real ones. (jPCA also tracks – panel B)
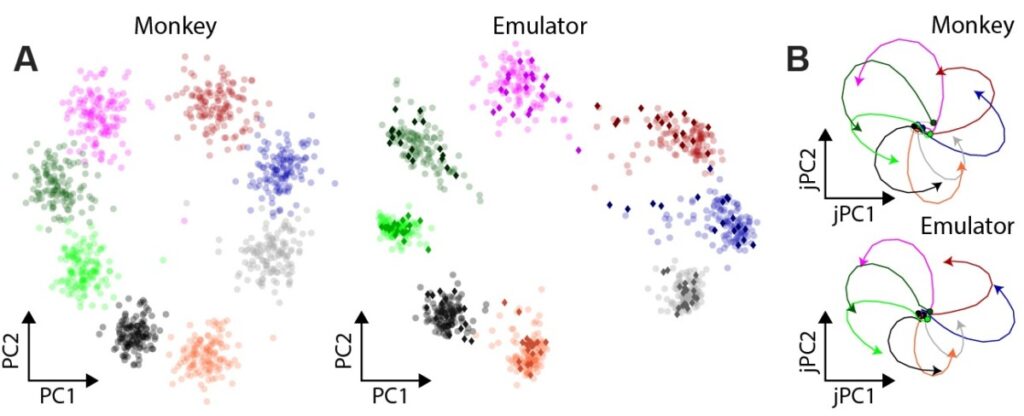
Since the synthetic neurons are faithful to the real ones, the problem of decoding synthetic neurons tracks the problem decoding real ones. Since jaBCI subjects have the same cursor control aptitude as invasive BCI users, we model the “learning in closed-loop problem” well also.
We hope the jaBCI will be a useful model for rapid prototyping of new (perhaps speculative) decoder designs that would not warrant the risk of a full BCI study. jaBCI can also increase our confidence in existing decoders by testing them in orders of magnitude more subjects.
Peeyush, A., et al. (2022). “Validation of a non-invasive, real-time, human-in-the-loop model of intracortical brain-computer interfaces.” Journal of Neural Engineering 19(5): 056038.