Vu, B.N., Bi, J., Wang, W., Huff, A., Kondragunta, S., Liu, Y. (2022). Application of geostationary satellite and high-resolution meteorology data in estimating hourly PM2.5 levels during the Camp Fire episode in California. Remote Sensing of Environment, 271, 112890.
Science Direct: Link
Particulate matter from wildland fire smoke can traverse hundreds of kilometers from where they originated and result in excess morbidity and mortality. However, estimating PM2.5 levels in and around wildland fires poses many challenges including lack of monitors at or near smoke plumes and temporally coverage since monitors generally obtain daily measurements yet smoke plumes continually change throughout the day due to wind. In this paper, we estimated total PM2.5 concentration during the Camp Fire episode, one of the deadliest wildland fires in California history. We used a random forest (RF) model to calibrate hourly regulatory monitor measurements (AQS maintained by the EPA) and low-cost sensors (PurpleAir) to Geostationary Operational Satellite-16 (GOES-16)’s AOD and fire spot detection variables, High-Resolution Rapid Refresh (HRRR) meteorology, and ancillary variables including land-use, distance to road, and a convolutional layer calculated for each hour. We tried three separate approaches: 1) an AQS-only model; 2) AQS + weighted PurpleAir model; and 3) AQS + weighted PurpleAir + SMOTE model. Synthetic Minority-Oversampling Technique (SMOTE) was applied to enhance and bolster the number of extremely high observations and improve model performance. The AQS-only model achieved and out of bag (OOB) R2 (RMSE) of 0.84 (12.00 μg/m3) and a spatial and temporal cross-validation (CV) R2 (RMSE) of 0.74 (16.28 μg/m3) and 0.73 (16.58 μg/m3), respectively. The AQS + weighted PurpleAir model’s OOB and spatial and temporal CV R2 (RMSE) was 0.86 (9.52 μg/m3), 0.75 (14.93 μg/m3), and 0.79 (11.89 μg/m3), respectively. The AQS + weighted PurpleAir + SMOTE model’s OOB and spatial and temporal CV R2 (RMSE) was 0.92 (10.44 μg/m3), 0.84 (12.36 μg/m3), and 0.85 (14.88 μg/m3), respectively. Results from this study indicate that increased measurements as well as the application of SMOTE not only improves model performance but also residual errors, and may aid in epidemiological studies investigating intense and acute exposure to wildland fire smoke.
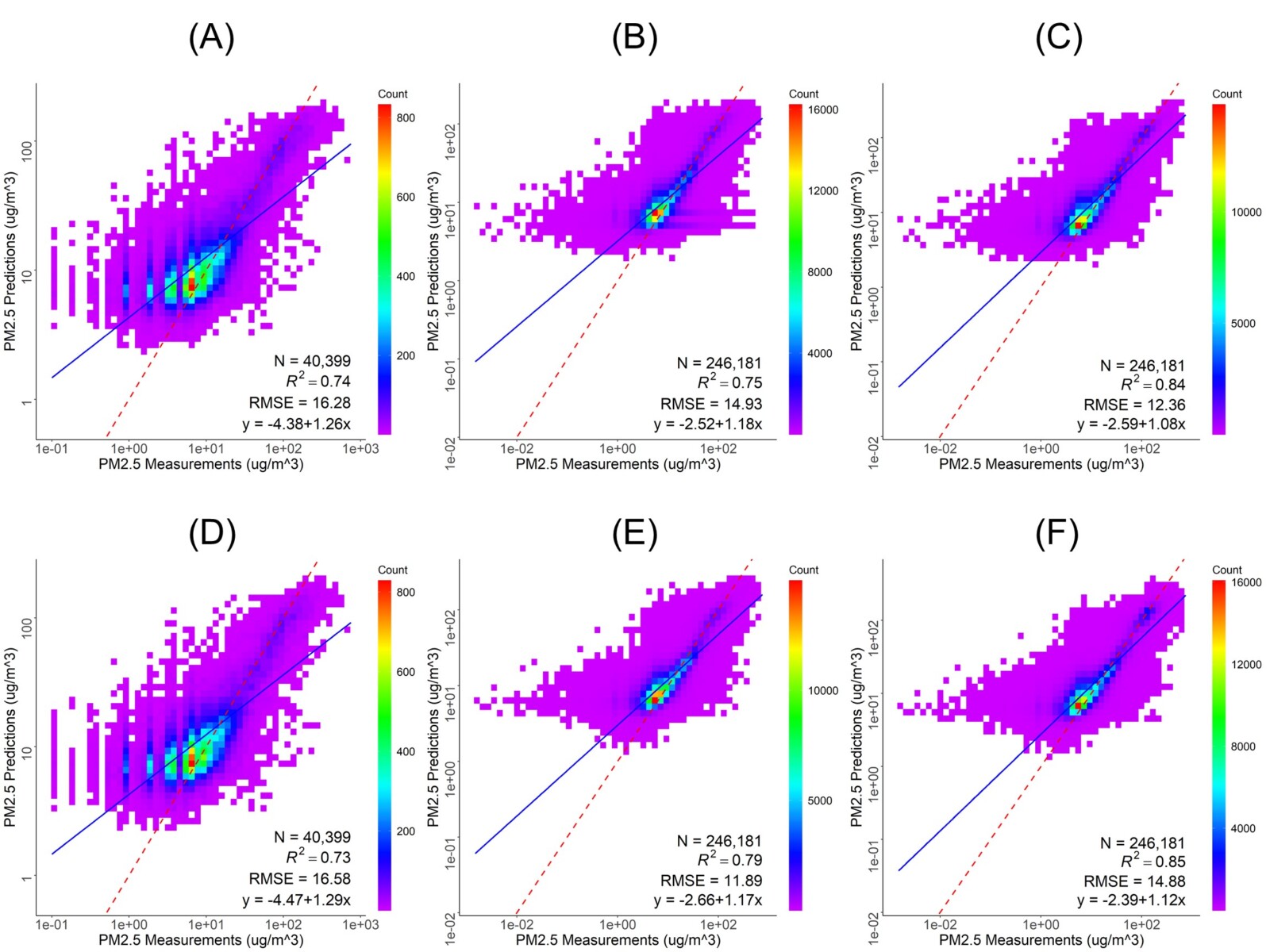
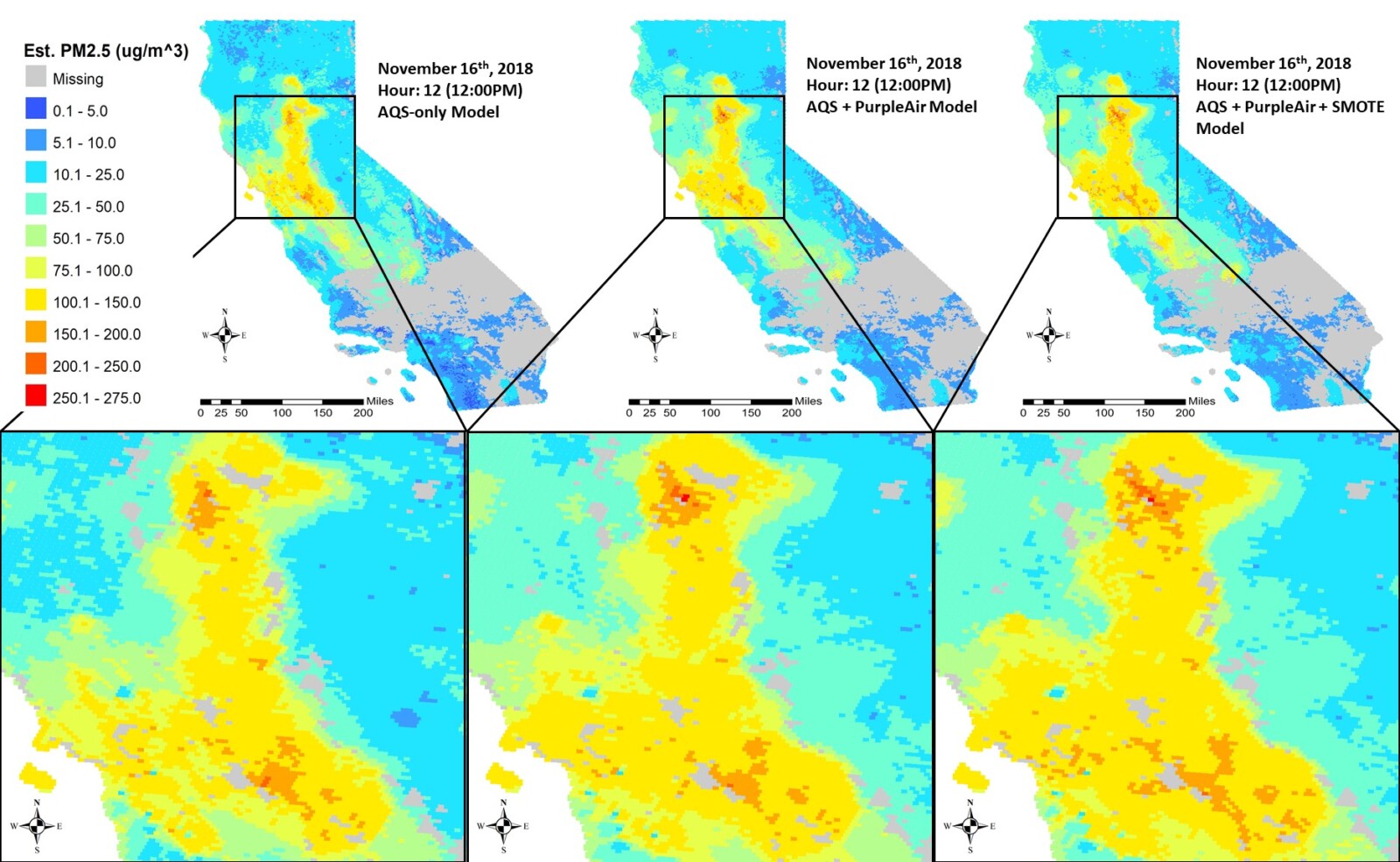
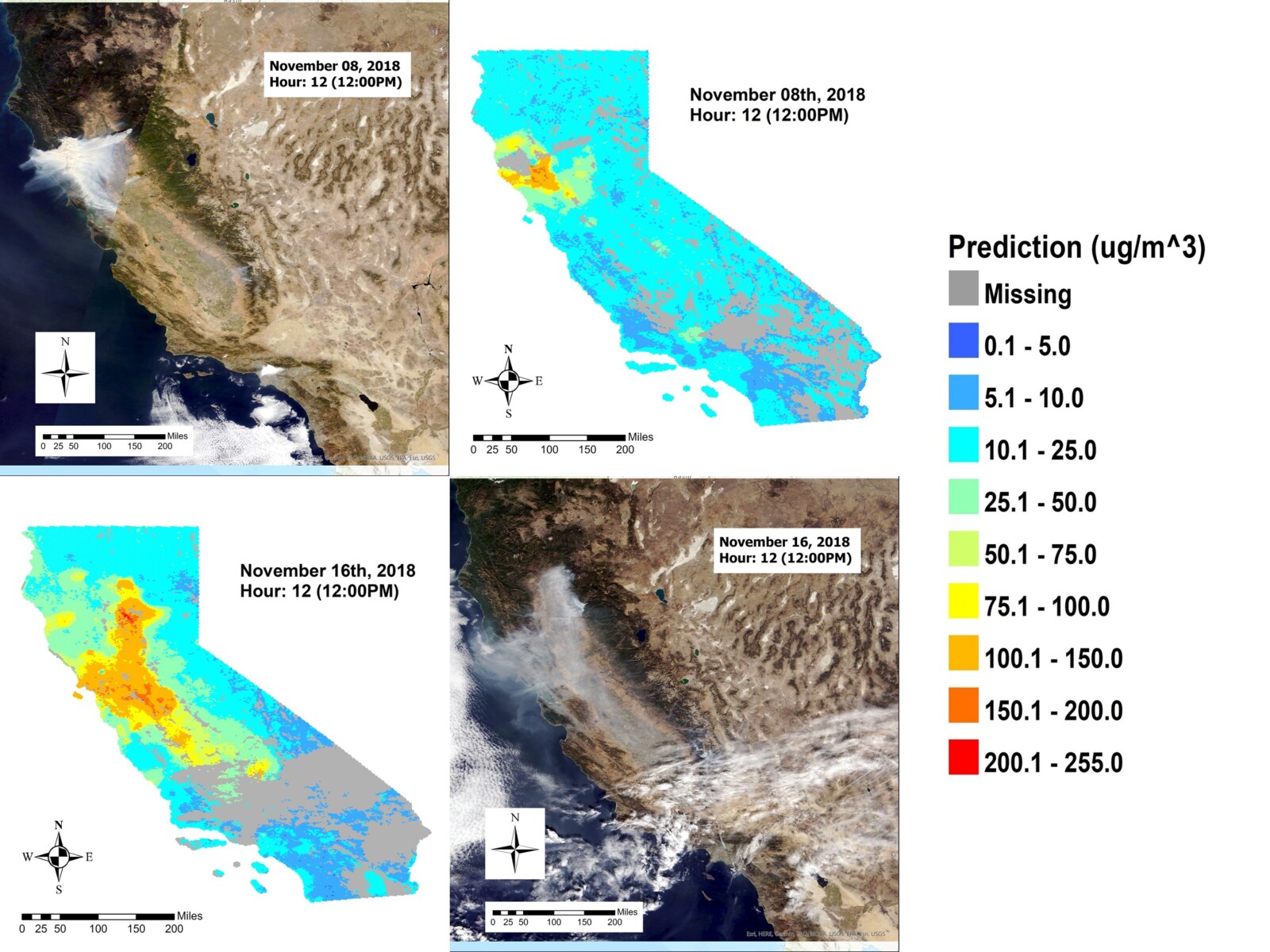